Have you ever tried to reproduce a recipe from your grandmother but couldn’t achieve the same result as her? If so, it may be a sign that your grandmother’s recipe is not easily replicable. In science, the same problem can arise with a study.
In 2016, the results of an online survey about the reproducibility of academic studies, completed by 1576 researchers, was published in the journal Nature (Baker, 2016). This survey revealed that 70% of respondents had already tried unsuccessfully to replicate the results of a study from another laboratory. It also found that more than 50% of the respondents had not been able to replicate certain results from their own laboratory. This type of observation has led a large proportion of researchers to speak of a “crisis of reproducibility” and to propose possible solutions to improve the reproducibility and the replicability of studies. It should be noted that these two terms are sometimes used interchangeably; in this blog post, we will favor the term replicability when it comes to replicating results from new data (unlike reproducibility, which is related to obtaining the same results from new analyses of the same dataset; Peels and Bouter, 2021).
Before addressing the possible solutions put forward by researchers to promote replicability, let’s start by distinguishing between different types of replicability (Goodman et al., 2016):
1. Replicability of the method
This type of replicability refers to the possibility of precisely replicating the method of a study thanks to the detailed information provided by the research team on the materials and procedures they used.
2. Replicability of results
Replicability of results refers to the ability to replicate the results of a study using a method as similar as possible to that of the original study.
3. Inferential replicability
Inferential replicability arises when a research team draws similar conclusions after reanalyzing data from a previously published study (which is connected to the concept of reproducibility), or in light of new results obtained from a replication study.
In our analogy with a cooking recipe, a low replicability of the method could arise if certain details were not written on the recipe (e.g., the exact quantity of each ingredient, the precise instruments used, etc.), which would make it difficult to follow the exact same steps as your grandmother. On the other hand, if the method of the recipe was sufficiently detailed and you followed all the steps to the letter without achieving the same results, this would be a case of low replicability of the results (that is, the capacity of the procedure to provide the same result). Finally, if you had achieved exactly the same result as your grandmother, but you judged that her recipe was more successful, this would be an issue of inferential replicability.
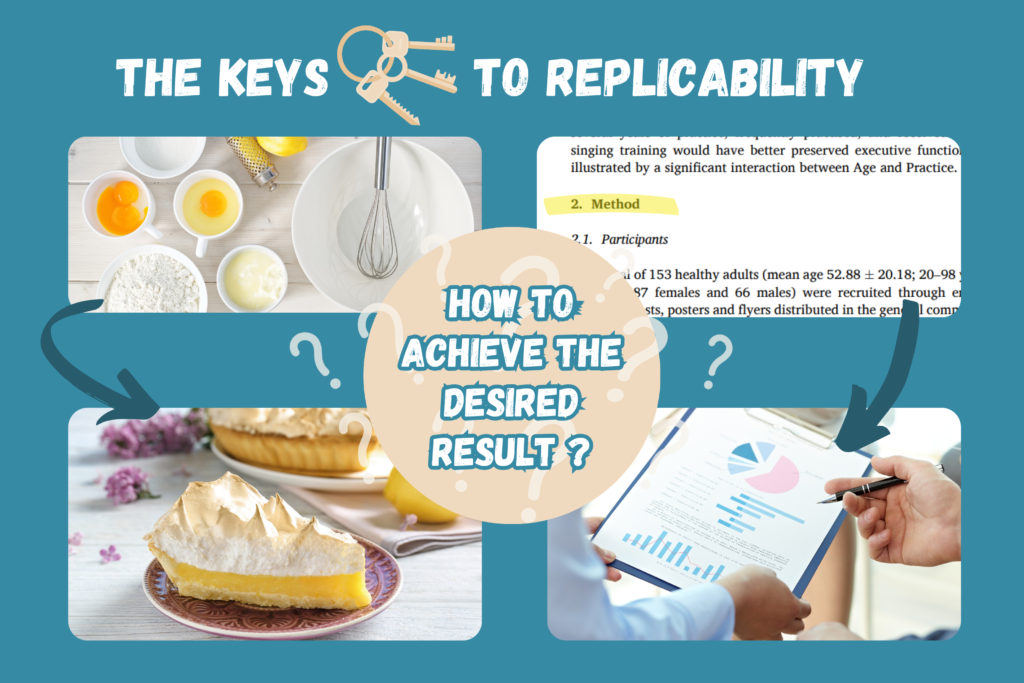
In psychology, biomedical sciences, and neurosciences, given the variability that exists in biological systems, including humans (e.g., differences between individuals in brain structure and function, in response to stress, etc.), expecting to obtain exactly the same results from one study to another is unrealistic. Nevertheless, the most salient results and the outline of the conclusion should be replicable (Begley & Ioannidis, 2015).
Several factors have been identified as potential causes of difficulties in reproducing or replicating study results (Baker, 2016; Begley & Ioannidis , 2015; Button et al., 2013). On the methodological level, these include a lack of result verification and quality control within the laboratory of origin (which notably reduce the risk of undetected errors affecting the results), a lack of transparency regarding the procedures used, shortcomings in terms of statistical analyses, as well as low statistical power, often linked to small sample sizes. This last point is an issue in neuroscience and neuroimaging studies, in particular because of the significant costs associated with data collection (e.g., for carrying out MRI examinations; Button et al., 2013) and in studies carried out with vulnerable populations, which are often more difficult to recruit (e.g., people suffering from neurodegenerative diseases). Even when sample sizes are large enough, an incomplete description of participant characteristics can hinder replicability. Indeed, if two studies have a supposedly similar population when, in reality, this is not the case (for example, one group is more educated or more active than the other), their results may differ. Finally, the fact that some specialized methodologies may lead to technical difficulties may also decrease reproducibility and replicability.
Organizational factors are also involved, such as the pressure to publish (the famous “publish or perish”), which is exacerbated by the strong competition to obtain research grants and positions in academia (Baker, 2016). In fact, valuing productivity (e.g., in terms of the number of scientific articles published) rather than quality and methodological rigor is detrimental to the reproducibility of published studies since it does not encourage rigor. Valuing innovative findings that generate excitement at the expense of establishing solid scientific fact through rigorous and incremental studies can eventually undermine scientific rigor (Begley & Ioannidis , 2015).
On a psychological level, confirmation bias can impair replicability (Begley & Ioannidis, 2015), especially inferential replicability. Confirmation bias consists in being too favorable to observations that support our hypotheses, to the detriment of those that contradict them. This bias can be at the origin of different conclusions made by two research teams based on similar results (but this can sometimes also be explained by other factors). Selective reporting, which is associated with confirmation bias and consists in presenting only a part of the results obtained (e.g., those which support our hypotheses), is a bad scientific practice which also harms the replicability of the results (Baker, 2016).
As the factors at the origin of issues with reproducibility and replicability are multiple, there is no single solution to remedy them. Fortunately, several possible solutions have been proposed and have been increasingly employed. These solutions involve several agents, including the various members of research teams, funding agencies, academic institutions, and scientific journals.
Solutions related to the training and supervision of research personnel
A first solution proposed by researchers (Begley & Ioannidis, 2015) is to adequately train students and research staff in methodology (e.g., experimental design, data collection and analysis, interpretation of results, etc.), metrology (i.e., all the methods and techniques used to obtain the greatest precision in measurements), and responsible conduct of research (on this subject, an online sensibilisation tool on the best practices in terms of responsible conduct of research is available on the website of the Fonds de recherche du Québec). Adequate methodological training may sometimes require collaborating with colleagues from other departments or universities with complementary expertise, who can provide mentorship to the student in training. Continuous training for researchers is also necessary given the constant methodological developments (Begley & Ioannidis, 2015). During the development of a project, quality control measurements must be put in place, including the verification of data and analyses, which constitute a safety net to detect potential errors and improve training as needed. The use of standardized protocols by team members also minimizes variability in the administration of procedures, thereby promoting replicability of the method and results within the team and beyond (Baker, 2016).
Solutions related to transparency and data sharing
For a study’s methodology to be replicable, enough detail about the procedures must be provided, which requires rigorous reporting of information (Goodman et al., 2016). It has been suggested that sharing videos to visualize the procedures could be a way to improve the replicability of the method (Pulverer, 2015). Additionally, scientific journals and institutions can encourage researchers to share raw data, analysis scripts, and materials used (such as visual or audio stimuli presented to participants in an experimental test; Gilmore et al., 2017). This information may be shared publicly on data repositories (e.g., Boréalis, OpenfMRI, NeuroVault). In the field of neuroimaging, the Organization for Human Brain Mapping (OHBM) published in 2016 a report on the best practices in analyzing and sharing data when using magnetic resonance imaging (Nichols et al., 2016).
When the data is shared publicly, it can be used in meta-analyses with a large amount of data (platforms such as BrainMap and NeuroSynth allow the generation of meta-analyses from MRI data). Meta-analyses measure the variability of results between studies, as well as the quality of the studies. Thus, the conclusions of meta-analyses are generally more robust than those of individual experimental studies.
Finally, a way to prevent the selective reporting of results is the pre-registration of scientific studies (Botvinik-Nezer & Wagner, 2022; Baker, 2016; Poldrack, 2018). Pre-registration consists in publishing your research protocol, including the hypotheses and analysis plans, even before the study is carried out. As the main and secondary measures to be analyzed are already specified by the team, it is expected that all the results concerning these measures will be published once the study has been carried out.
Solutions related to a paradigm shift valuing quality over quantity
As part of the process of awarding research grants and scholarships, not only the quality of the studies but also the efforts of the researchers in terms of transparency and reproducibility should be taken into account (Begley & Ioannidis, 2015). For example, the adoption of randomization measures, blinding procedures (when possible and appropriate), and good statistical practices should be valued. Greater weight should also be given to research questions and methods, rather than to the results obtained (Poldrack et al., 2018).
Scientific journals could also issue guidelines for good scientific practices and checklists for authors when submitting articles (Pulverer, 2015; Baker, 2016). Moreover, academic institutions can reward the completion of replicable and robust studies and provide continuing education opportunities for researchers and students. Such actions are likely to improve the quality of scientific publications and their replicability (Begley & Ioannidis, 2015; Baker, 2016).
Researchers are of the opinion that publishers of scientific journals should also encourage the implementation of confirmatory studies and the publication of negative results, i.e., results that do not support the initial hypotheses and do not replicate the expected results (Wagenmakers & Forstmann, 2014; Ioannidis, 2006). If multiple research teams unsuccessfully attempt to replicate one research team’s results, but their negative results are not published, this fuels the reproducibility crisis. Indeed, if negative results were published more often, teams could decide to look into other research questions rather than persisting in reproducing or replicating the same studies without success. Moreover, a certain skepticism about new results, which are often published in the most reputable journals, is desirable (Begley & Ioannidis, 2015). Indeed, any new result must be reproduced several times before it can be considered “true”.
It is therefore joint actions, at several levels and involving different agents of research, that are necessary to promote the reproducibility and replicability of scientific studies. As several of the actions that can be carried out at the level of the research teams (e.g., better training and supervision of personnel, establishment of systematic verification procedures within the team, etc.) are costly in terms of human and financial resources, the support from granting agencies, academic institutions and publishers of scientific journals is essential!
References:
Baker, M. (2016). 1,500 scientists lift the lid on reproducibility. Nature, 533(7604), 452-454. https://doi.org/10.1038/533452a
Begley, C. G., & Ioannidis, J. P. (2015). Reproducibility in science: improving the standard for basic and preclinical research. Circ Res, 116(1), 116-126. https://doi.org/10.1161/CIRCRESAHA.114.303819
Botvinik-Nezer, R., & Wager, T. D. (2022). Reproducibility in Neuroimaging Analysis: Challenges and Solutions. Biol Psychiatry Cogn Neurosci Neuroimaging. https://doi.org/10.1016/j.bpsc.2022.12.006
Button, K. S., Ioannidis, J. P., Mokrysz, C., Nosek, B. A., Flint, J., Robinson, E. S., & Munafo, M. R. (2013). Power failure: why small sample size undermines the reliability of neuroscience. Nat Rev Neurosci, 14(5), 365-376. https://doi.org/10.1038/nrn3475
Gilmore, R. O., Diaz, M. T., Wyble, B. A., & Yarkoni, T. (2017). Progress toward openness, transparency, and reproducibility in cognitive neuroscience. Ann N Y Acad Sci, 1396(1), 5-18. https://doi.org/10.1111/nyas.13325
Goodman, S. N., Fanelli, D., & Ioannidis, J. P. (2016). What does research reproducibility mean? Sci Transl Med, 8(341), 341ps312. https://doi.org/10.1126/scitranslmed.aaf5027
Ioannidis, J.P. (2006). Journals should publish all “null” results and should sparingly publish “positive” results. Cancer Epidemiol Biomarkers Prev, 15:186. doi: 10.1158/1055-9965.EPI-05-0921.
Poldrack, R. A. (2019). The Costs of Reproducibility. Neuron, 101(1), 11-14. https://doi.org/10.1016/j.neuron.2018.11.030
Pulverer, B. (2015). Reproducibility blues. EMBO J, 34(22), 2721-2724. https://doi.org/10.15252/embj.201570090
Wagenmakers, E. J., & Forstmann, B. U. (2014). Rewarding high-power replication research. Cortex, 51, 105-106. https://doi.org/10.1016/j.cortex.2013.09.010