The importance of replication in science
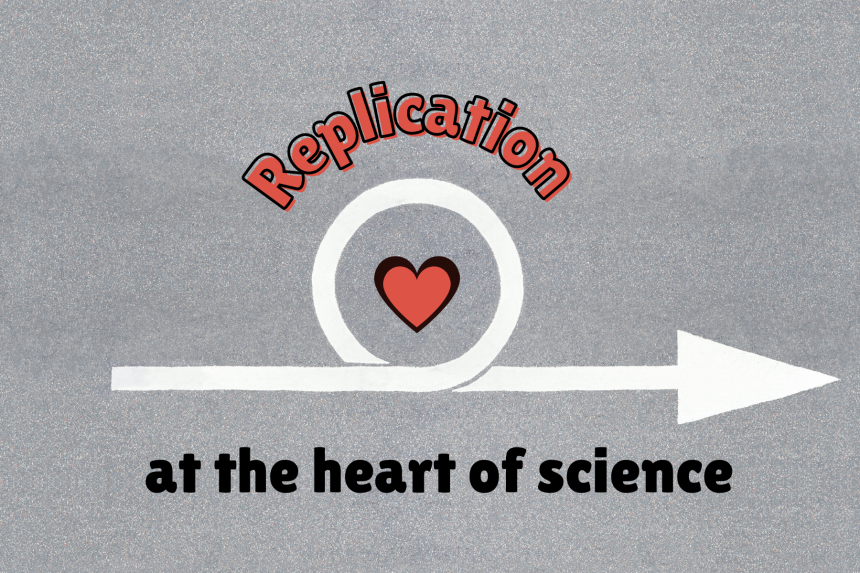
Can strong conclusions be drawn from a single study? If two studies contradict each other, does this mean that we cannot trust science?
The answer is no.
Several factors can explain differences in results from one study to another, such as differences in the study sample, the method used or the analyzes performed. Let’s take a fictional example with two studies focusing on the effectiveness of musical training to improve the ability to perceive speech in the presence of environmental noise. If the results of only one of the two studies made it possible to conclude that the training was effective, this could be explained by differences in the age of the people recruited in the two studies, differences in the frequency, intensity or duration of the training, the precision of the tests used to measure progress, etc.
To better understand these phenomena and generate more robust conclusions than those provided by a single study, science relies on an essential process: replication.
The goal of replication is to advance current theories and knowledge by confronting them with new evidence (Nosek and Errington, 2020; KNAW, 2018). Replication makes it possible to lend credibility to scientific assertions, theories, hypothesis, or models, when the results that led to their formulation are reproduced; this is especially strong if replications originate from several independent research teams across different countries. When the results of one study are contradicted by the results of one or several other studies, confidence in the results of the original study should be limited. Research teams must try to understand what explains the differences in results and carry out additional studies to verify the results of the first study. Researchers may also be required to propose modifications to existing theories and models or to create new ones, which better explain all of the results (Nosek and Errington, 2020).
A replication study can be defined as “an independent repetition of a previously published study, performed under similar circumstances and using similar methods” (KNAW, 2018).
However, the degree of replication of an original study can vary, as shown on the continuum in Figure 1.
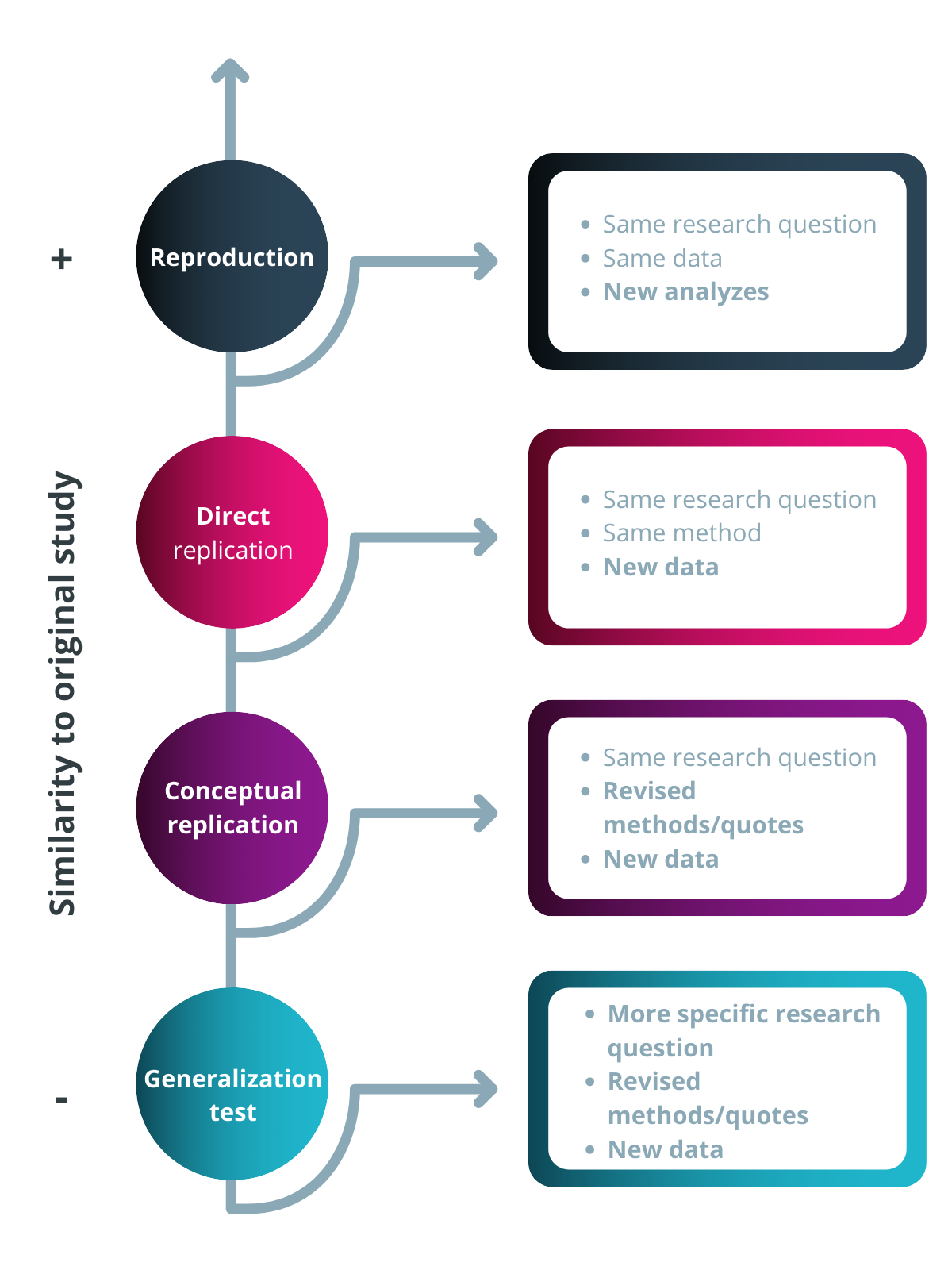
Figure 1. Diagram illustrating the different degrees of replication of an original study.
Reproduction is a type of replication that involves reanalyzing data that has already been collected. The new analysis can be exactly the same and thus make it possible to see if errors have been made (this type of replication can be done within the same laboratory, by another member of the team). An alternative or improved analysis plan can also be used and thus make it possible to verify whether the original results are robust (Peels and Bouter, 2021).
Direct replication involves collecting new data, but using as much of the same method as the original study. When direct replication leads to the same results as the original study, it is less likely that the results of the original study are attributable to chance, error, or specificity of the original sample, for example. This type of replication can thus support the accuracy of the results of the original study. It is particularly useful when the hypothesis or models tested are new (Peels and Bouter, 2021).
Conceptual replication is a type of study that involves collecting new data to answer the same research question as the original study, but making some changes to the design or experimental method. If the results are reproduced, this suggests that the methodological variations do not influence the original results. The level of confidence granted to the original results is increased (Peels and Bouter, 2021).
When scientific knowledge accumulates and the understanding of a phenomenon improves, it becomes possible to test increasingly specific hypotheses, and to verify under what conditions (e.g., with which populations) the original results are valid. This can be done through generalization tests (Nosek and Errington, 2020). In this case, research teams replicate portions of the study, but make methodological changes to answer a more specific research question.
Replication studies and generalization tests identify conditions under which results can be generalized and expected, but also conditions under which results are not valid (Nosek & Errington, 2020). Let’s go back to our example from the beginning, where research teams studied the effectiveness of musical training (let’s call it ABC) to improve the ability to perceive speech in the presence of noises (see Figure 2).
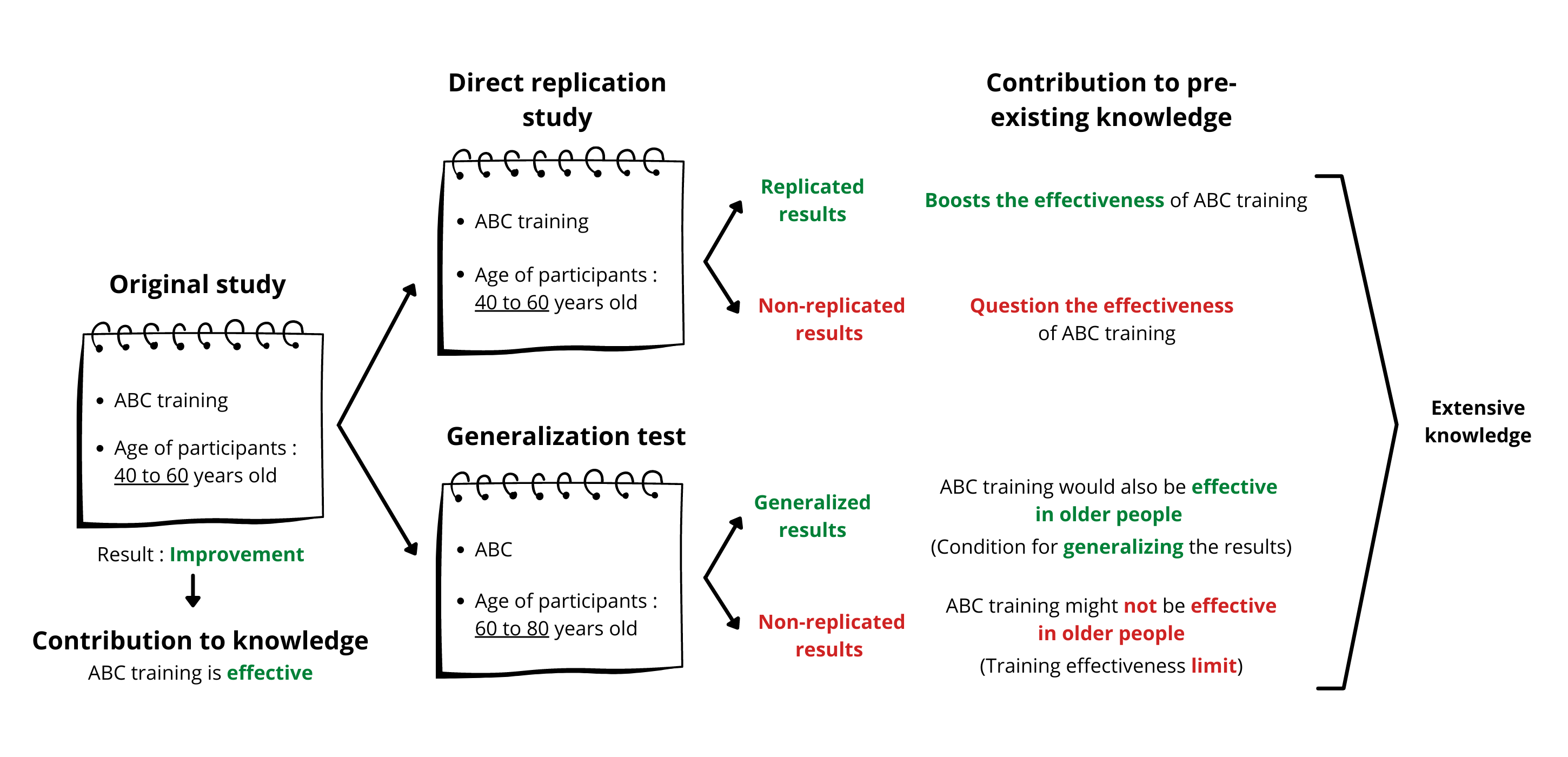
Figure 2. Illustration of the contribution of replication and generalization tests to the advancement of scientific knowledge.
The direct replication study would use the same method as Study 1 (in which ABC training was reported to be effective in improving the ability to perceive speech in noise in people aged 40-60). If the original study is replicated, the hypothesis that the ABC treatment is effective will be strengthened. If the original study is not replicated, the hypothesis will be called into question. In both cases, carrying out additional studies would make it possible to confirm or refute the conclusions of the original study. In fact, even when research teams attempt to use exactly the same method, differences can arise. For example, studies can be conducted in different historical and environmental contexts (such as in times of pandemic, geopolitical conflict or inflation). Studies conducted in different countries or regions are also likely to recruit people with different socio-demographic or cultural characteristics, education levels and linguistic background. However, all these differences can influence the results and lead to divergent conclusions. Replication studies can help identify the influence of certain factors on the results that may have been previously unsuspected. And even when two studies are carried out in contexts and with very similar samples, differences related to the methodological quality of the studies or to chance may arise. The result of the original study could itself be due to chance! This is why several—even many—studies are needed to answer a research question.
In the generalization test given as an example in Figure 2, the same training as in the original study was used, but with people aged 60 and 80 years old. If an improvement was observed following training, this would suggest that the results of the first study are generalizable to another population, i.e., older people. If there was no improvement, this would suggest that training is not effective in older people. In this case, although the results seem to be in contradiction with those of the original study, they most likely would be explained by methodological differences. However, it remains possible that the training would not have worked even in younger adults, but that question cannot be resolved with this study.
In short, in this example, the results of the replication and generalization tests contribute to the advancement of scientific knowledge—whether or not they supported those of the original study—in particular by specifying the conditions for effective training. This knowledge is important to better predict the contexts in which a training benefit could be expected.
In reality, the results are often complex to interpret, since different studies can differ at multiple levels. Replication is therefore an essential tool for scientists. It is the pooling of the results of several studies that makes it possible to generate robust and nuanced conclusions. The pooling of the results of several studies is best carried out within the framework of systematic reviews and meta-analyses, which are useful scientific tools for dissecting all the studies carried out on a question and drawing more substantial and robust conclusions.
In conclusion, when innovative results are published, it is important to wait until other studies have been published on the subject before drawing definitive conclusions. It is also expected and explainable that some of the studies obtain contradictory results. It is the sum of the observations which, over time, will provide increasingly clear and robust answers!